Course Outcomes | After the lecture, the participants are expected to: 1. Understand data and the concepts behind it. 2. Identify different types of data and their examples. 3. Categorize data into machine-readable or non-machine-readable format. 4. Recognize benefits of transitioning from paper to digital surveys. 5. Determine factors needed to be considered when collecting data digitally. 6. Define sampling methods and identify sampling biases to avoid when doing digital surveys. 7. Understand how to protect data by abiding with data privacy and security. 8. Recognize the concept and importance of data quality in decision making. 9. Understand the different aspects of data quality. 10. Conduct a data quality assessment using the tool. 11. Recognize the concepts and importance of data audit or maturity assessment in better institutionalization of data culture. 12. Understand the pillars, components, and relevant indicators for evaluating data maturity 13. Conduct a data maturity assessment using the tool. |
Outline/ Content | 1. Data Concepts 1.1 Definition of Data 1.2 Different Types of Data 1.3 Examples of Data 2. Digital Data Collection 2.1 Digital vs. Paper-based Collection 2.2 Factors to Consider in Digital Data Collection 2.3 Type of Sampling Biases 2.4 Protecting Data Collected 3. Data Quality 3.1 What and Why of Data Quality 3.2 Data Quality Dimensions 3.3 Data Quality Assessment 4. Data Audit 4.1 What and Why of Data Audit or Data Maturity Assessment 4.2 Data Maturity Assessment Framework 4.3 Rapid Data Maturity Assessment Tool 4.4 Levels of Data Maturity 4.5 Improving the Level of Data Maturity |
Lecturers
![]() ![]() ![]() ![]() |
Jeddahlyn Gacera is a Data Associate of Action for Economic Reforms. She worked as an ETL consultant for 5 years and is currently a Data Scientist at Accenture. There, she collaborates with and delivers data-driven solutions to a leading financial services provider in the country, and one of the biggest platform companies in the world. She has a bachelor’s degree in Mathematics from University of the Philippines Diliman, and a master’s degree in Data Science from the Asian Institute of Management. Dr. Laurence Go is a Fellow of Action for Economic Reforms and currently the Coordinator of AER’s Data-Driven Development Program. He previously worked on data solutions for the COVID-19 Action Network and AER’s Food and Agriculture Policy and Fiscal Policy Programs. He also worked with WeSolve to study COVID-19 procurement processes in the Philippines. He obtained his economics PhD at the Wharton School, University of Pennsylvania and is currently a postdoctoral researcher at the Autònoma de Barcelona. He leads the Development Data Lab of both COLLABDev and PH3D, a project co-financed by the European Union. JC Peralta is a Data Associate of Action for Economic Reforms and a climate and data scientist in the energy industry. He received his Masters in Atmospheric Science from ADMU in 2019 and has worked extensively with climate big data with focus on the local dynamics and impacts of regional monsoons over the Philippines and in Southeast Asia. He has been selected as one of the mentors in the Oxford School of Climate Change 2021 and has also worked on various projects in disaster science, renewable energy, transport networks, data for social good, and climate action advocacies. Daffodil Santillan is a Data Associate of the PH3D Project co- funded by the Action for Economic Reforms and the European Union. She is currently pursuing a master’s degree in Development Economics at the School of Economics, University of the Philippines Diliman. Previously, she served as a Chief of Staff at the Social Security System (SSS) for five years. Her research interests are in economic growth & government, social welfare & income redistribution. |
Recommended Readings | Data Basics by Coursera https://www.snapsurveys.com/blog/great-debate-paper-surveys-online-surveys/ Health Knowledge. 2021. Methods of sampling from a population. https://www.healthknowledge.org.uk/public-health-textbook/research-methods/1a-epidemiology/methods-of-sampling-population Medium. 2021. Here’s our Visual Guide to Digital Data Collection (in the time of COVID19). https://medium.com/pollicy/heres-our-visual-guide-to-digital-data-collection-in-the-time-of-covid19-95aebc8fdc5c#:~:text=Digital%20data%20collection%20is%20the,tablets%2C%20and%20other%20digital%20devices. Shona McCombes.2019. An introduction to sampling methods. https://www.scribbr.com/methodology/sampling-methods/ Data Quality Assessment. Leo L. Pipino, Yang W. Lee, and Richard Y. Wang. April 2002/Vol. 45, No. 4ve COMMUNICATIONS OF THE ACM UN Global Marketplace Data Quality Assessment Handbook Data Quality Assessment Checklist (DQA) by USAID Learning Lab https://usaidlearninglab.org/library/data-quality-assessment-checklist-dqa |
Course Manual
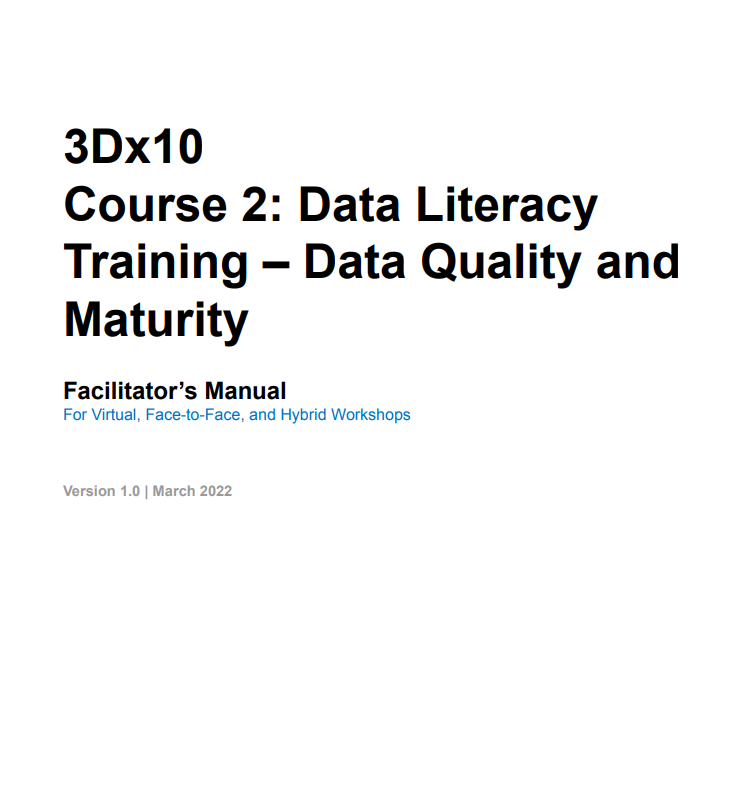
3Dx10
Course 2:
Data Literacy Training –
Data Quality and Maturity
Facilitator’s Manual
For Virtual, Face-to-Face, and Hybrid Workshops
Download Review Notes In Filipino

Recommended Readings
Data Basics by Coursera
https://www.coursera.org/lecture/probability-intro/data-basics-Q0zu3
DeFranzo, W., 2021. The Great Debate: Paper Surveys vs. Online Surveys. https://www.snapsurveys.com/blog/great-debate-paper-surveys-online-surveys/
Health Knowledge. 2021. Methods of sampling from a population. https://www.healthknowledge.org.uk/public-health-textbook/research-methods/1a-epidemiology/methods-of-sampling-population
Medium. 2021. Here’s our Visual Guide to Digital Data Collection (in the time of COVID19). https://medium.com/pollicy/heres-our-visual-guide-to-digital-data-collection-in-the-time-of-covid19-95aebc8fdc5c#:~:text=Digital%20data%20collection%20is%20the,tablets%2C%20and%20other%20digital%20devices.
Shona McCombes.2019. An introduction to sampling methods. https://www.scribbr.com/methodology/sampling-methods/
Data Quality Assessment. Leo L. Pipino, Yang W. Lee, and Richard Y. Wang. April 2002/Vol. 45, No. 4ve COMMUNICATIONS OF THE ACM
UN Global Marketplace Data Quality Assessment Handbook
Data Quality Assessment Checklist (DQA) by USAID Learning Lab
https://usaidlearninglab.org/library/data-quality-assessment-checklist-dqa